Following Patient Trajectories to Prioritize High Risk Patients
Neural Ordinary Differential Equations (Neural-ODE) are continuous deep learning architectures that create dynamic models that can model time series data. Latent ODEs in particular may be helpful for irregularly sampled time series data, which occur in many applications, particularly in healthcare. One challenge is modeling differences in condition, or state, that can be at baseline or represent a change in condition. One approach to address severe discontinuities is the use of event functions, which allow the ODE to be terminated with a criteria is satisfied and then resume solving the ODE with the new state. However, this is not necessarily reflective of real-world data, where changes in state may influence the trajectory but not represent a complete discontinuity. We propose explicitly separating time series data that represent continuous values (dynamic data), and time series data that reflect a specific condition at baseline or during the time interval (conditional data). Separate autoencoders are used to create separate latent spaces that are used to initialize the Neural ODE and then trained together with a loss calculated between the decoded Neural ODE output and the original values.
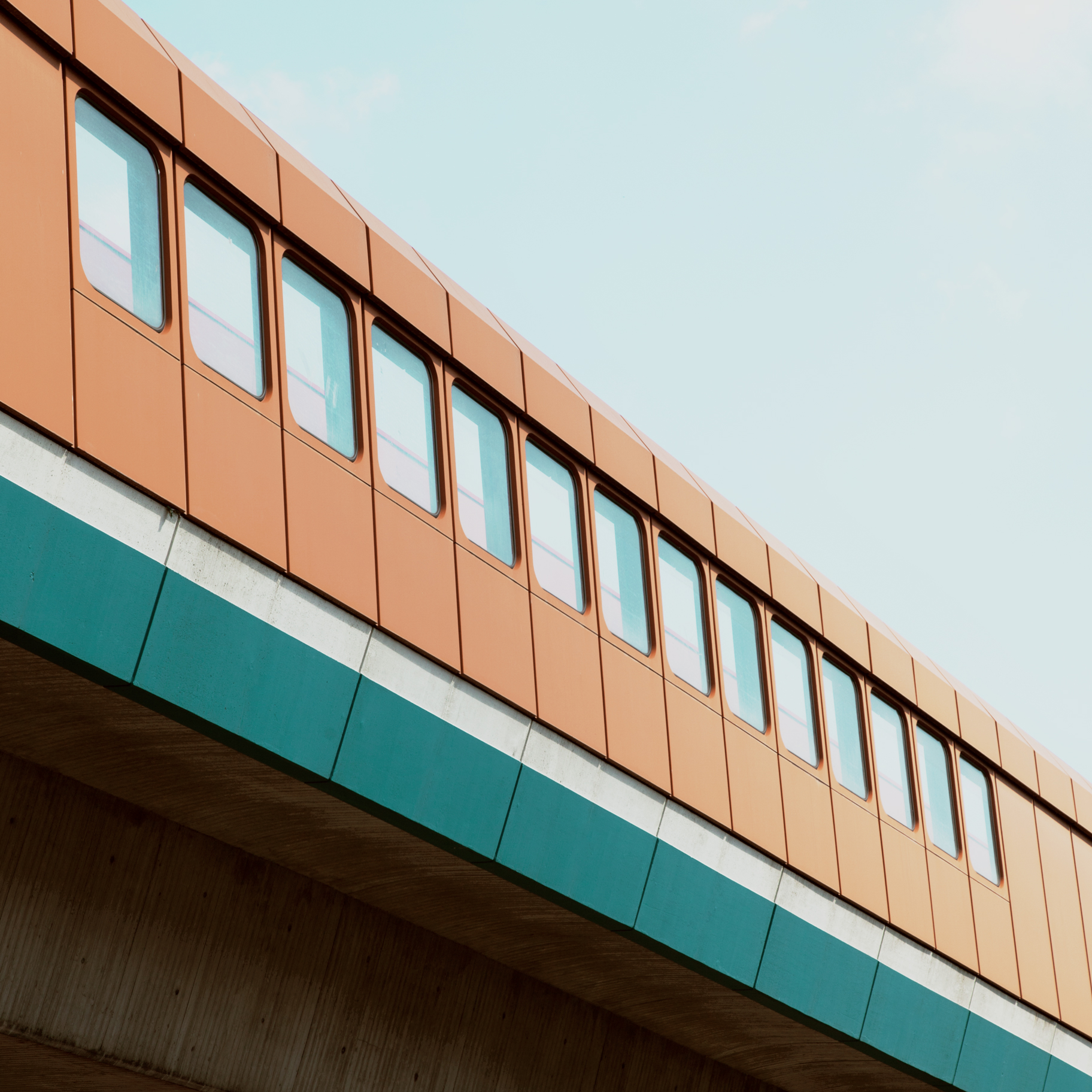